联系编辑部
电话:0371-63387308
0371-65330928
邮箱:guoshuxuebao@caas.cn
汇款信息
地址:郑州市航海东路南中国
农业科学院郑州果树研究所
收款人:《果树学报》编辑部
邮编:450009
银行汇款(审稿费、版面费)
开户行:农行管城区支行
账号:16045601040002497
开户单位:中国农业科学院郑
州果树研究所
附言注明第一作者姓名和稿号
期刊服务
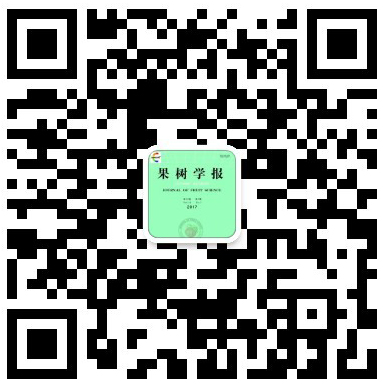
扫描二维码,关注微信号
访问量
- 今日访问:
- 昨日访问:
- 本月访问:
- 总访问:
本期栏目 优先出版
|
刊出日期:2025-2-10 |
- DOI:10.13925/j.cnki.gsxb.20240344
- 全文:
PDF(下载次数:9) 摘要(点击次数:9)
- DOI:10.13925/j.cnki.gsxb.20240409
- 全文:
PDF(下载次数:9) 摘要(点击次数:9)
- DOI:10.13925/j.cnki.gsxb.20240491
- 全文:
PDF(下载次数:10) 摘要(点击次数:10)
- DOI:10.13925/j.cnki.gsxb.20240566
- 全文:
PDF(下载次数:5) 摘要(点击次数:5)
- DOI:10.13925/j.cnki.gsxb.20240538
- 全文:
PDF(下载次数:9) 摘要(点击次数:9)
- DOI:10.13925/j.cnki.gsxb.20240567
- 全文:
PDF(下载次数:20) 摘要(点击次数:20)
- DOI:10.13925/j.cnki.gsxb.20240510
- 全文:
PDF(下载次数:12) 摘要(点击次数:12)